The manufacturing industry today is marked by its pronounced shift toward digitization.
While this transition promises enhanced efficiency and accuracy, it also unearths critical challenges, especially when it comes to maintaining impeccable product quality. A minor oversight on a circuit board or a subtle software bug could jeopardize your entire production batch, tarnishing your brand’s image in the process.
According to the International Federation of Robotics, global robot installations were projected to grow by 10% in 2022, reaching almost 570,000 units, with average annual growth rates expected in the medium to upper single-digit range from 2022 to 2025. As the adoption of robotics and automation intensifies, it becomes increasingly critical for businesses to support these systems with advanced data analytics to ensure impeccable product quality.
Strategies for Implementing Advanced Data Analytics and Automation
Overcoming Human Limitations
In manufacturing quality control, overcoming human limitations is a critical objective. Human inspectors are susceptible to fatigue-induced errors, and the subjectivity in standardizing quality assessment among inspectors can lead to inconsistencies. To address these challenges, manufacturers are turning to cutting-edge technologies, such as Computer Vision (CV) and Advanced Machine Learning algorithms.
CV and Advanced Machine Learning offer a revolutionary approach to quality control. These systems ensure precise, consistent, and rapid anomaly detection, surpassing the capabilities of human inspectors. Unlike humans, these technologies do not tire, ensuring round-the-clock vigilance over manufacturing processes. Moreover, they excel in standardizing quality assessment by applying consistent criteria to every inspection, eliminating subjectivity. Beyond recognizing superficial defects, these algorithms excel in diagnosing intricate issues that might escape the human eye, making them indispensable tools for ensuring impeccable product quality in the manufacturing industry.
Ditching Outdated QC Methods
Despite the era of Industry 4.0, many manufacturing companies still rely on outdated quality control methods, including spreadsheets, paper logs, and manual checklists. These archaic approaches not only consume excessive time but are also prone to errors. To stay competitive and ensure the highest quality standards, transitioning to an integrated digital quality management system is essential. Such automated systems enable real-time monitoring, data analysis, and quality assurance, reducing the risks associated with manual processes. These systems typically consist of the following components:
Data Integration: Digital quality management systems integrate with various data sources, including production equipment, sensors, and databases, to collect real-time data on product quality and manufacturing processes.
Automation: These systems automate quality control tasks, such as inspections and data collection, reducing the reliance on manual labor and minimizing human errors.
Quality Assurance: There are modules in these digital systems for quality assurance, allowing organizations to define quality standards, track compliance, and manage deviations.
Integration with IoT: Many modern systems integrate with the Internet of Things (IoT) devices and sensors, allowing for continuous data collection from production equipment and products.
Scalability: These systems are designed to be scalable, accommodating the needs of growing manufacturing operations.
Harnessing Actionable Insights from Big Data
In a digitized manufacturing environment, every process, machine, and product generates a wealth of data. However, raw data, without the lens of insightful analytics, can cloud decision-making. This is where advanced data analytics and artificial intelligence (AI) come into play. By employing these technologies, businesses can derive actionable intelligence from the data deluge. This shift toward data-driven decision-making enables a proactive approach to quality management, where potential issues are identified and addressed even before they manifest, preventing costly defects and production delays.
As a pivot to this technological overhaul, integrating low-code/no-code platforms can simplify the implementation of these advanced systems. For instance, a no-code platform can be used to swiftly design dashboards for real-time quality monitoring without delving deep into intricate coding. This ensures that even those unfamiliar with programming can contribute to maintaining quality standards.
A Case Study in Advanced Data Analytics and AI Integration
One notable example of the application of advanced data analytics and AI in manufacturing quality control is the case of Global Unichip Corporation (GUC). As GUC develops increasingly complex microchips, ensuring quality at scale becomes a formidable challenge. To address this, GUC collaborated with proteanTecs, an AWS Partner Network member, to implement a solution that combines embedded data within the ASICs (application-specific integrated circuits) with predictive AI. This approach allows GUC to track and proactively repair silicon defects before they can lead to system failure. The results of this partnership have been remarkable, with GUC and proteanTecs significantly enhancing the quality and reliability of GUC’s microchips.
The integration of predictive AI into quality management exemplifies the power of data-driven decision-making in the manufacturing industry. By identifying potential issues in advance and taking corrective actions promptly, manufacturers can not only prevent costly defects but also optimize production processes for greater efficiency and consistency. This highlights the transformative potential of advanced data analytics and AI in ensuring impeccable product quality.
Final Thoughts
The manufacturing industry’s pursuit of the highest quality standards necessitates the integration of advanced data analytics, automation, and low-code/no-code solutions. Manufacturers that embrace these technologies not only enhance their operational efficiency but also fortify their market reputation. As the era of Industry 4.0 unfolds, staying ahead in quality control is not just an option but a strategic imperative for sustained success in the manufacturing sector.
Read more:
How to optimize manufacturing quality through advanced data analytics and automation
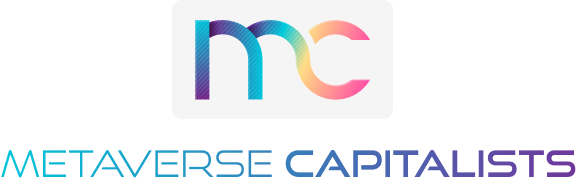